At EasyTechJunkie, we're committed to delivering accurate, trustworthy information. Our expert-authored content is rigorously fact-checked and sourced from credible authorities. Discover how we uphold the highest standards in providing you with reliable knowledge.
What Are Expert System Shells?
In expert systems, expert system shells are the software containing an interface, an inference engine, and the formatted skeleton of a knowledge base. In essence, an expert system shell is an empty bowl to be filled with the expert knowledge elements that the inference engine may process for users. Expert systems are computer applications that provide problem-solving help for specific problems that a user might need to access to solve, for example, a utility software difficulty in operation. A knowledge engineer would use this shell to develop the knowledge base and customize it to meet the needs of its particular client base of users. It would be customized to take a user’s input and interpret that information to the data repository and, by comparison, locate matching information that might help guide the user to a solution.
Along with the control information that is deposited into a knowledge base, are rules and attributes definitions that govern the release of information to users. The knowledge base is constructed of statements of expertise that mimic the analysis process of a human expert in pursuit of enough knowledge to achieve a solution. Expert system shells must provide capabilities to bolster the knowledge engineer’s job in the development of a knowledge base that may operate as a real time expert system. In such an expert system, the base may be in constant data change by deletions or additions of data because industrial systems, networks, hardware, and software systems change over time. This constant change of data input from other management systems must not falter the base’s ability to reason at the same expert level, regardless of changes.
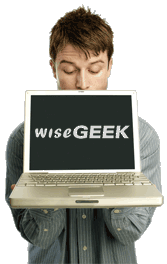
Expert systems shells provide the bare bones for imitation of human expert reasoning in rule methods known as forward chaining and backward chaining. Forward chaining in these shells enables taking data from a user and using inference engine rules to locate more data relative to that information until there is enough information to form a conclusion. Because the initial data received is what drives the seeking, this method is called a data driven method. An application that illustrates this forward-chaining method might explore the possibilities of arrangement of components within a computer to arrive at the best placement of the components.
Backward chaining gathers data only as it needs it when a knowledge base is being queried on a consultation. It has the goal of finding a value for C and reasons backward to discover the value of A and B that conclude the goal value of C. This method of reasoning from present data to prior data that was the underpinning of present data is called goal-driven method. An application illustrating expert system shells rules of inference might include a medical doctor inputting a current set of symptoms for background information on the same or similar symptoms in background information from a particular medical diagnosis expert system.
Inferred knowledge is gained by examination of existing facts to arrive at likely new information. This is the reasoning process that inhabits the inference engine in expert system shells. This process is what initiates the forward or backward chaining in rule-based expert systems. Inference rules that build the inference engines in expert system shells are made up of conditional “if” clauses and “then” clauses in ruling statements that facilitate guidance of steps. These steps might be in fields of financial services, human resources, and mortgage loan handling, among others, to try to discover rules of thumb as probable recommendations when a definitive answer is not possible.
AS FEATURED ON:
AS FEATURED ON:


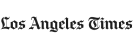

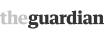
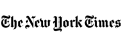
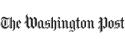


Discuss this Article
Post your comments